Tutorial: Doing Grad-CAM on the Food-Non-Food (FNF) model¶
This is a tutorial on how to perform Grad-CAM on the FNF model using the
XAI.FNFGradCAM()
class that is provided in this library.
Summary of the recommended workflow:
Instantiate the desired FNF model and load its desired pretrained weights.
Create a PyTorch dataset using the
XAI.utils.datasets.DatasetFromCSV()
class.Instantiate the
XAI.FNFGradCAM()
object.Do the Grad-CAM algorithm on the dataset created in Step 2 using the
do_gradcam()
function.If necessary, plot and save the Grad-CAM results using the
plot_gradcam()
function.
There will be 2 ways that you can perform thm Grad-CAM on the FNF model, both of which will be covered in this tutorial:
To begin, activate the virtual environment that has the XAI
library
installed and import the library.
1 | import XAI
|
Method 1: Using the imported XAI
library¶
Step 1: Load the desired PyTorch model¶
This tutorial assumes that this step is already completed and that the desired
PyTorch model is loaded into the model
variable. Refer to this tutorial if
necessary.
Step 2: Creating the dataset¶
This tutorial assumes that this step is already completed and that the dataset
of images is created as the ds
variable. Refer to
Tutorial: Creating a DatasetFromCSV Dataset for a walkthrough on how to create the dataset.
The dataset ds
used in this tutorial contains 10 images.
Step 3: Instantiate FNFGradCAM()
class object¶
Instantiate the XAI.FNFGradCAM()
class by doing the following. Here, the
target layer used in the Grad-CAM computation is conv2d_7b
.
1 2 3 4 | fnf_gc = XAI.FNFGradCam(
model=model,
target_layer_name="conv2d_7b",
)
|
The fnf_gc
object has the following 3 attributes:
>>> fnf_gc.device
device(type='cuda', index=0)
>>> fnf_gc.target_layer_name
'conv2d_7b'
>>> fnf_gc.model
Step 4: Do the Grad-CAM using the do_gradcam()
function¶
This function does the following 3 observable things:
Computes the Grad-CAM attribution map for each image and saves each map as a numpy array (
.npy
file) in the specified output folder (output_folder
).Returns a Pandas DataFrame containing the results of performing Grad-CAM and saves this DataFrame as
gradcam_metadata.csv
inoutput_folder
.Plots and saves the Grad-CAM numpy arrays as
.png
files ifplot_gradcam=True
.
The following folder structure will be created in output_folder
to save the
3 outputs listed above:
output_folder/
├── gradcam_metadata.csv
│
├── gradcam_numpy_arrays/
│ ├── 164c728e-86d2-11eb-b774-06d7ab6752a4.npy
│ ├── 164c728f-86d2-11eb-b774-06d7ab6752a4.npy
│ ├── ...
│ └── 164c72a5-86d2-11eb-b774-06d7ab6752a4.npy
│
└── gradcam_plots/ <--- This folder will be created if `plot_gradcam=True`
├── 164c728e-86d2-11eb-b774-06d7ab6752a4.png
├── 164c728f-86d2-11eb-b774-06d7ab6752a4.png
├── ...
└── 164c72a5-86d2-11eb-b774-06d7ab6752a4.png
Note:
The dataset passed into the function is
ds
.The function performs Grad-CAM on batches of images of size
batch_size
.The ReLU function can be included or excluded in the Grad-CAM computation by setting
relu_attributions
to eitherTrue
orFalse
respectively. Refer to Tutorial: How to Interpret Grad-CAM Results for FoodDX Models to understand the purpose of the ReLU function. It is recommended to set this as ``False``.If
return_metadata=True
, each batch of images will be forward-passed into the model to get the model’s predicted probabilities for each image. These probabilities will be included in the returned DataFrame.
You can refer to the documentation on the FNFGradCAM.do_gradcam()
function
for an explanation of each argument.
1 2 3 4 5 6 7 8 | fnf_results_df = fnf_gc.do_gradcam(
image_dataset=ds,
batch_size=2,
relu_attributions=False,
return_metadata=True,
output_folder="/output_folder",
plot_gradcam=False
)
|
Working on batch 1 of 5, batch_size = 2...
Working on batch 2 of 5, batch_size = 2...
Working on batch 3 of 5, batch_size = 2...
Working on batch 4 of 5, batch_size = 2...
Working on batch 5 of 5, batch_size = 2...
The returned DataFrame (fnf_results_df
) will look like the following:
>>> fnf_results_df
id |
target_layer |
label_fnf |
path_fns |
path_gradcam |
path_gradcam_plot |
pred_proba_0 |
pred_proba_1 |
---|---|---|---|---|---|---|---|
164c729b-86d2-11eb-b774-06d7ab6752a4 |
conv2d_7b |
1 |
path/to/original/image/164c729b-86d2-11eb-b774-06d7ab6752a4.png |
output_folder/gradcam_numpy_arrays/164c729b-86d2-11eb-b774-06d7ab6752a4.npy |
nan |
0.000242 |
0.999758 |
… |
… |
… |
… |
… |
… |
… |
… |
If
return_metadata=False
, thepred_proba_0
andpred_proba_1
columns will benan
values.If
plot_gradcam=True
, thepath_gradcam_plot
column will be populated with the paths to the plotted.png
images.
Step 5: Plot Grad-CAM results using plot_gradcam()
, if necessary¶
It is also possible to call the plot_gradcam()
function separately to plot
and save the Grad-CAM numpy arrays into .png
images. The plot_gradcam()
function takes in the DataFrame returned from the do_gradcam()
function,
retrieves the necessary information from the columns of the DataFrame to plot
the images.
At this point, it is important to understand that plotting,
showing (aka printing) and saving the images are independent of each other.
While plot_gradcam()
always plots the images, whether or not the
images are shown inline and/or saved as a .png
file is dependent on the
savefig
and plt_show
arguments.
If
savefig=True
andplt_show=True
, the plots will both be shown inline and saved as a.png
file to the folder specified insavefig_dir
.If
savefig=True
andplt_show=False
, the plots will not be shown inline but will be saved as a.png
file to the folder specified insavefig_dir
. Vice versa.If
savefig=False
andplt_show=False
, the plots will not be saved and shown inline. It is expected that this case is unhelpful and will not be used.
The following folder structure will be created in savefig_dir
where the
images will be saved. Here, you can see that if the same folder is used for the
do_gradcam()
and plot_gradcam()
functions, we will end up with the
folder structure that is shown here.
output_folder/
├── gradcam_metadata.csv <--- If `save_csv=True`, this file will be created and saved.
│
└── gradcam_plots/
├── 164c728e-86d2-11eb-b774-06d7ab6752a4.png
├── 164c728f-86d2-11eb-b774-06d7ab6752a4.png
├── ...
└── 164c72a5-86d2-11eb-b774-06d7ab6752a4.png
You can refer to the documentation on the FNFGradCAM.plot_gradcam()
fuction
for an explanation of each argument.
1 2 3 4 5 6 7 | fnf_results_df = fnf_gc.plot_gradcam(
do_gradcam_df=fnf_results_df,
savefig_dir="/output_folder",
savefig=True,
plt_show=True,
save_csv=True
)
|
Below is a sample of the output from the plot_gradcam()
function that you
should expect to see.
Plotting Grad-CAM results for 164c729b-86d2-11eb-b774-06d7ab6752a4...
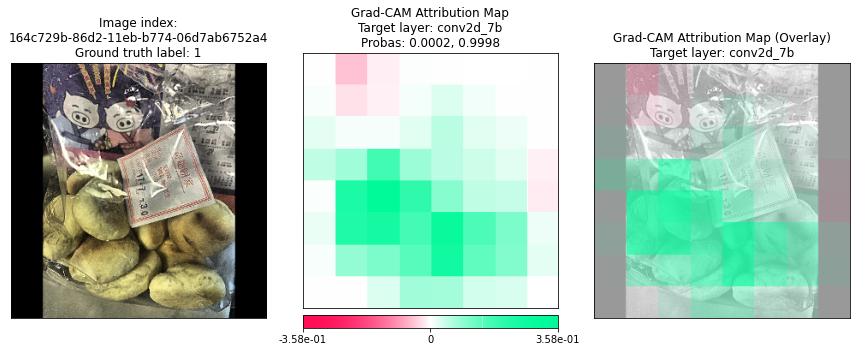
Plotting Grad-CAM results for 164c72a5-86d2-11eb-b774-06d7ab6752a4...
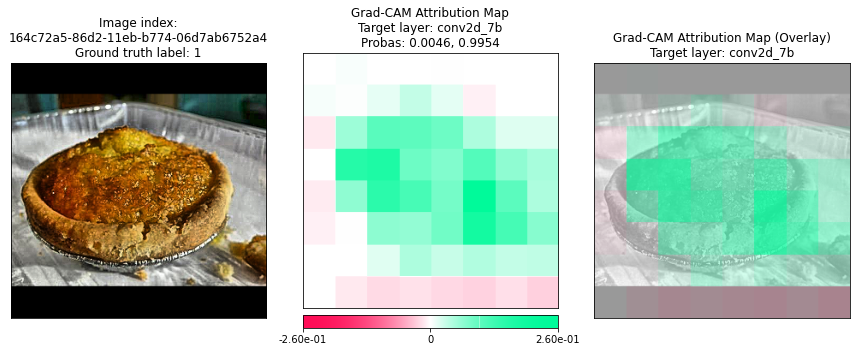
Method 2: Using the Command Line Interface (CLI) Tool¶
A CLI is the second option that can be used to perform Grad-CAM on the FNF
model. Unlike Method 1, the CLI does not
require the user to manually execute
Step 1 and
Step 2 before running the
do_gradcam()
and plot_gradcam()
functions. This is because the CLI
executes the above 5 steps all together in a single call.
To get an overview of the CLI tool and its arguments, refer to Tutorial: Overview of the Command Line Interface (CLI).
Assuming that we are running the CLI tool from the following parent folder…
.
├── data/
│ ├── data.csv
│ └── images/
│ ├── 164c728e-86d2-11eb-b774-06d7ab6752a4.png
│ ├── 164c728f-86d2-11eb-b774-06d7ab6752a4.png
│ ├── ...
│ └── 164c72a5-86d2-11eb-b774-06d7ab6752a4.png
├── checkpoints/
│ └── epoch_104.pth
└── otuput_folder/
we will have the following paths to the arguments of the CLI tool:
path_to_csv = ./data/data.csv
pretrained_weights_folder = ./checkpoints/epoch_104.pth
output_folder = ./output_folder
To maintain consistency with Method 1, we will still be:
Using
conv2d_7b
as the target layerbatch_size=2
relu_attributions=False
return_metadata=True
plot_gradcam=True
To perform Grad-CAM on the FNF model, run the following in the command line:
$ XAI FNF ./data/data.csv ./checkpoints ./output_folder conv2d_7b --batch_size 2 --return_metadata --plot_gradcam
Arguments passed to the parser:
-------------------------------
fnf_or_fs : FNF
path_to_csv : ./data/data2.csv
pretrained_weights_folder : ./checkpoints
output_folder : ./output_folder
target_layer_name : conv2d_7b
batch_size : 2
relu_attributions : False
return_metadata : True
plot_gradcam : True
About the dataset:
------------------
Number of images: 10
Batch size: 2
Running the XAI library:
------------------------
Application : Food-Non-Food (FNF)
Number of model(s) loaded : 1
Working on batch 1 of 5, batch_size = 2...
Working on batch 2 of 5, batch_size = 2...
Working on batch 3 of 5, batch_size = 2...
Working on batch 4 of 5, batch_size = 2...
Working on batch 5 of 5, batch_size = 2...
Plotting Grad-CAM results for 164c729b-86d2-11eb-b774-06d7ab6752a4...
Saving 164c729b-86d2-11eb-b774-06d7ab6752a4 plot to ./output_folder/gradcam_plots/164c729b-86d2-11eb-b774-06d7ab6752a4.png
Plotting Grad-CAM results for 164c72a5-86d2-11eb-b774-06d7ab6752a4...
Saving 164c72a5-86d2-11eb-b774-06d7ab6752a4 plot to ./output_folder/gradcam_plots/164c72a5-86d2-11eb-b774-06d7ab6752a4.png
...
Similar to Method 1, the Grad-CAM results
will be saved in output_folder
as follows:
.
├── data/
│ ├── data.csv
│ └── images/
│ ├── 164c728e-86d2-11eb-b774-06d7ab6752a4.png
│ ├── 164c728f-86d2-11eb-b774-06d7ab6752a4.png
│ ├── ...
│ └── 164c72a5-86d2-11eb-b774-06d7ab6752a4.png
├── checkpoints/
│ └── epoch_104.pth
└── otuput_folder/
└── gradcam_metadata.csv
│
├── gradcam_numpy_arrays/
│ ├── 164c728e-86d2-11eb-b774-06d7ab6752a4.npy
│ ├── 164c728f-86d2-11eb-b774-06d7ab6752a4.npy
│ ├── ...
│ └── 164c72a5-86d2-11eb-b774-06d7ab6752a4.npy
│
└── gradcam_plots/
├── 164c728e-86d2-11eb-b774-06d7ab6752a4.png
├── 164c728f-86d2-11eb-b774-06d7ab6752a4.png
├── ...
└── 164c72a5-86d2-11eb-b774-06d7ab6752a4.png